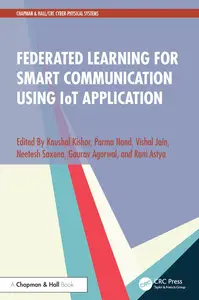
Free Download Federated Learning for Smart Communication using IoT Application (Chapman & Hall/CRC Cyber-Physical Systems) by Kaushal Kishor, Parma Nand, Vishal Jain
English | October 30, 2024 | ISBN: 1032788127 | 274 pages | MOBI | 6.05 Mb
The effectiveness of federated learning in high‑performance information systems and informatics‑based solutions for addressing current information support requirements is demonstrated in this book. To address heterogeneity challenges in Internet of Things (IoT) contexts, Federated Learning for Smart Communication using IoT Application analyses the development of personalized federated learning algorithms capable of mitigating the detrimental consequences of heterogeneity in several dimensions. It includes case studies of IoT‑based human activity recognition to show the efficacy of personalized federated learning for intelligent IoT applications.