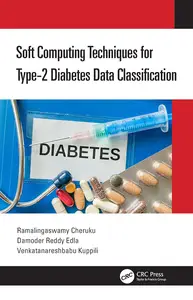
Free Download Soft Computing Techniques for Type-2 Diabetes Data Classification
English | 2020 | ISBN: 0367236540 | 169 Pages | PDF EPUB (True) | 14 MB
Diabetes Mellitus (DM, commonly referred to as diabetes, is a metabolic disorder in which there are high blood sugar levels over a prolonged period. Lack of sufficient insulin causes presence of excess sugar levels in the blood. As a result the glucose levels in diabetic patients are more than normal ones. It has symptoms like frequent urination, increased hunger, increase thirst and high blood sugar. There are mainly three types of diabetes namely type-1, type-2 and gestational diabetes. Type-1 DM occurs due to immune system mistakenly attacks and destroys the beta-cells and Type-2 DM occurs due to insulin resistance. Gestational DM occurs in women during pregnancy due to insulin blocking by pregnancy harmones. Among these three types of DM, type-2 DM is more prevalence, and impacting so many millions of people across the world. Classification and predictive systems are actually reliable in the health care sector to explore hidden patterns in the patients data. These systems aid, medical professionals to enhance their diagnosis, prognosis along with remedy organizing techniques. The less percentage of improvement in classifier predictive accuracy is very important for medical diagnosis purposes where mistakes can cause a lot of damage to patient’s life. Hence, we need a more accurate classification system for prediction of type-2 DM. Although, most of the above classification algorithms are efficient, they failed to provide good accuracy with low computational cost. In this book, we proposed various classification algorithms using soft computing techniques like Neural Networks (NNs), Fuzzy Systems (FS) and Swarm Intelligence (SI). The experimental results demonstrate that these algorithms are able to produce high classification accuracy at less computational cost. The contributions presented in this book shall attempt to address the following objectives using soft computing approaches for identification of diabetes mellitus.